A new study challenges the perception of AI’s diagnostic prowess, suggesting that multiple-choice benchmarks may paint an overly optimistic picture. Re-evaluating the MedQA dataset, researchers discovered a significant drop in accuracy when AI models, including Gemini 2.5 Pro, were tasked with generating free-text diagnoses instead of selecting from pre-defined answers. Gemini 2.5 Pro, for example, achieved a 95.5% accuracy rate in multiple-choice scenarios, but this fell to 91.5% when generating free-text. This disparity highlights the ‘back-engineering’ effect, where AI models leverage provided options to achieve higher scores. The study underscores the necessity of employing free-text benchmarks to more accurately assess AI’s diagnostic capabilities, mirroring the cognitive demands of real-world medical reasoning. The research team advocates for a shift away from multiple-choice protocols to prevent an inflated view of AI’s true potential in medical diagnosis. Further discussion can be found on Reddit: https://old.reddit.com/r/artificial/comments/1kktczs/reevaluating_medqa_why_current_benchmarks/
AI Diagnostic Abilities: Free-Text Tests Expose Overstated Performance in Multiple-Choice Benchmarks
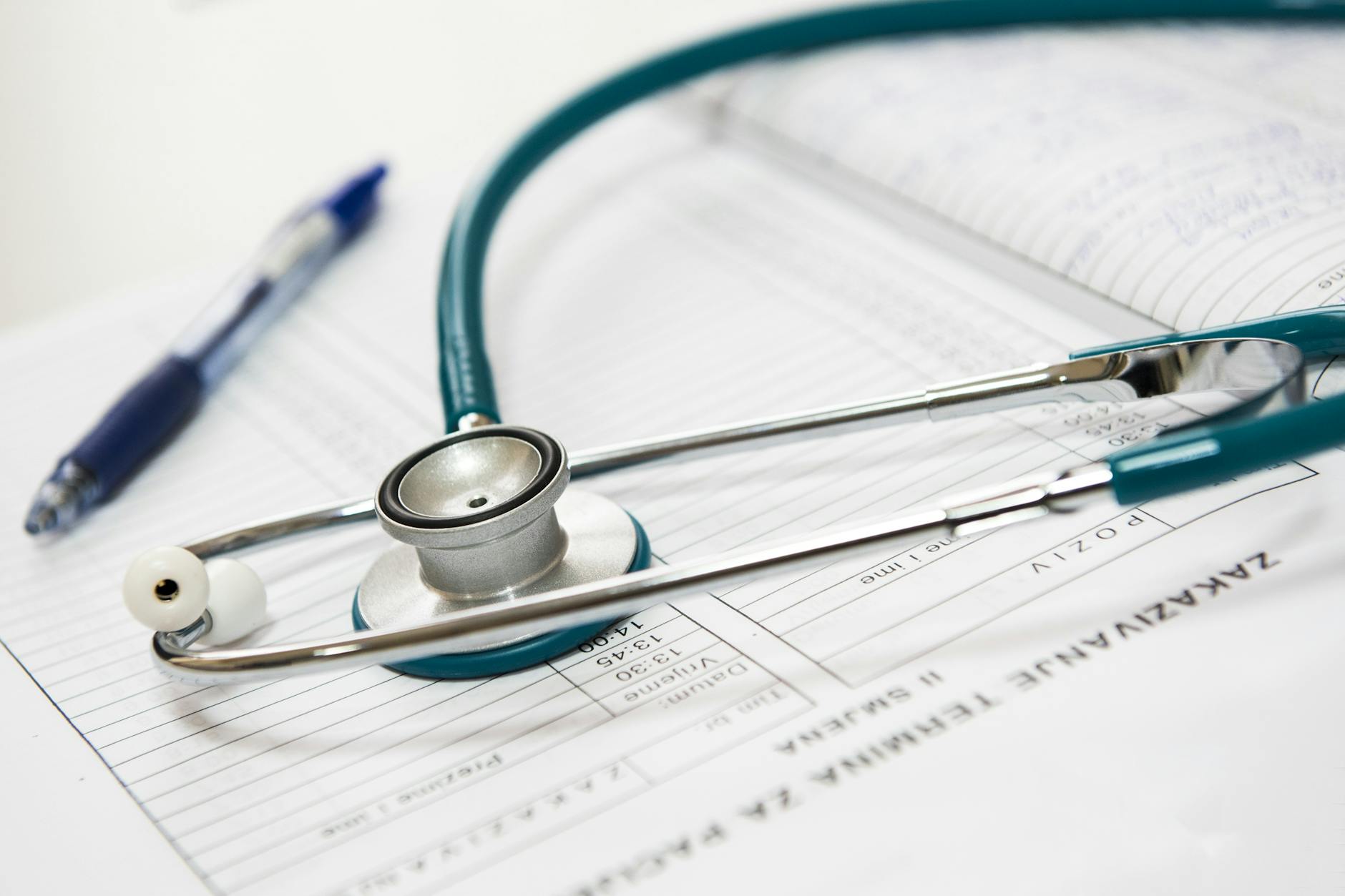