A computer science student is tapping into the expertise of machine learning and MLOps professionals to understand the data challenges hindering the efficiency of LLM pipelines. Seeking practical insights, the student is focusing on identifying common data bottlenecks experienced after model training, including difficulties in data collection, cleaning, labeling, and monitoring for drift. Furthermore, the student’s research delves into the effect of techniques like Reinforcement Learning from Human Feedback (RLHF) and synthetic data generation on the ongoing demand for relevant, domain-specific data. The inquiry also explores the obstacles encountered when sourcing data from challenging domains like finance, healthcare, log analytics, and multi-modal applications. The student is particularly interested in learning which workflow tasks professionals believe are ripe for automation to alleviate these bottlenecks. This crowdsourced research initiative originated from a Reddit post by user /u/kritnu, inviting contributions from the wider ML/MLOps community.
CS Student Investigates Data Bottlenecks Plaguing Machine Learning Pipelines
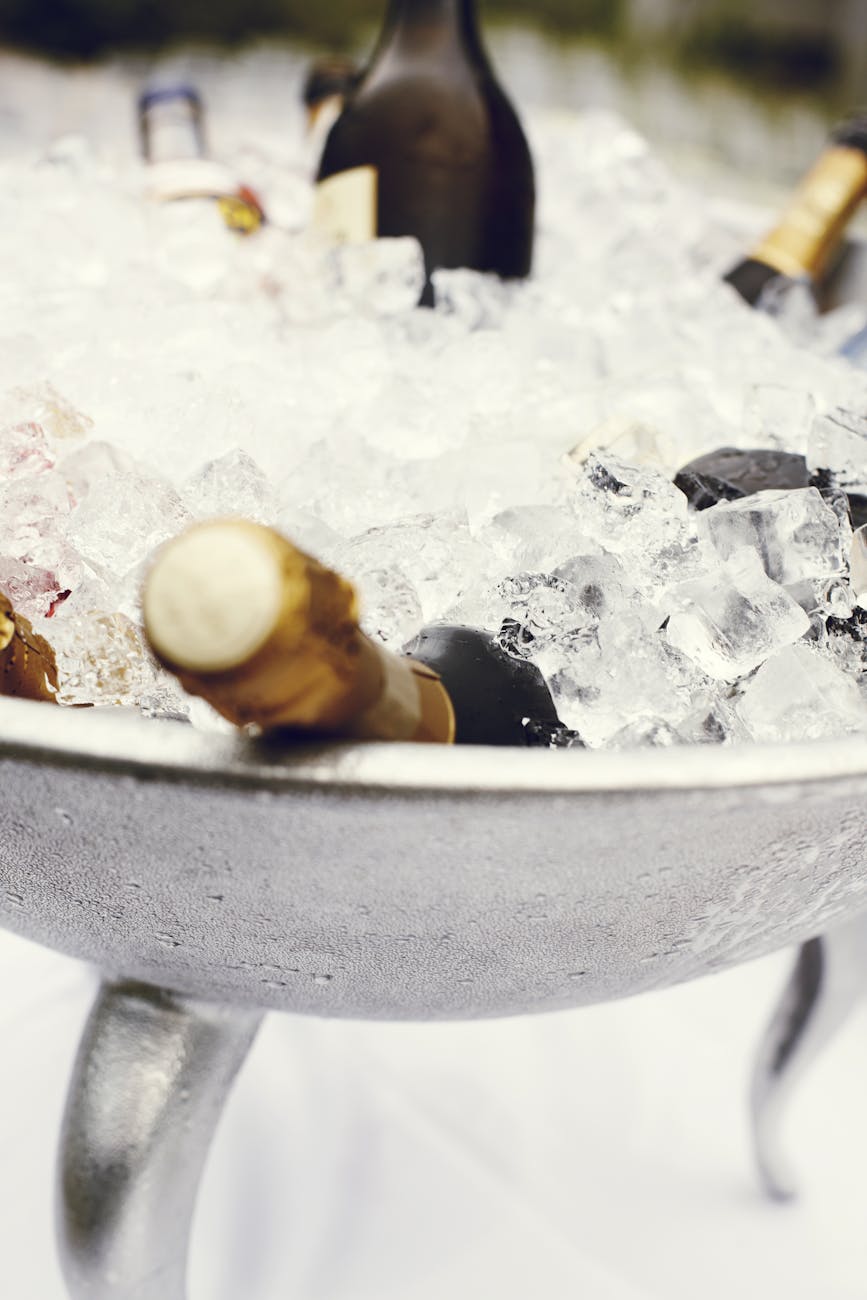